AI in Financial Services: Key Insights from the 2024 Cognaize Survey
by Cognaize on Nov 25, 2024 12:02:29 PM
Artificial Intelligence (AI) continues to play an increasingly pivotal role in transforming industries, particularly those managing large volumes of data, complex compliance demands, and intricate decision-making processes. A recent survey targeting professionals across various sectors provides valuable insights into the current and anticipated applications, challenges, and needs associated with AI. This article explores the main takeaways from the survey and sheds light on how AI can address the specific needs of financial and data-heavy industries while navigating practical obstacles.
AI in the Financial Sector: Major Players and Use Cases
The survey reflects significant participation from financial services, including commercial and central banking, underlining the financial sector’s ongoing interest in leveraging AI for enhanced productivity and decision-making. The concentration of responses from these domains suggests that banking and financial institutions are heavily invested in exploring AI’s potential, both for strategic advantages and for operational efficiency.
Among specific use cases, financial spreading, fraud detection, and credit risk assessment were highlighted as top areas for AI application.
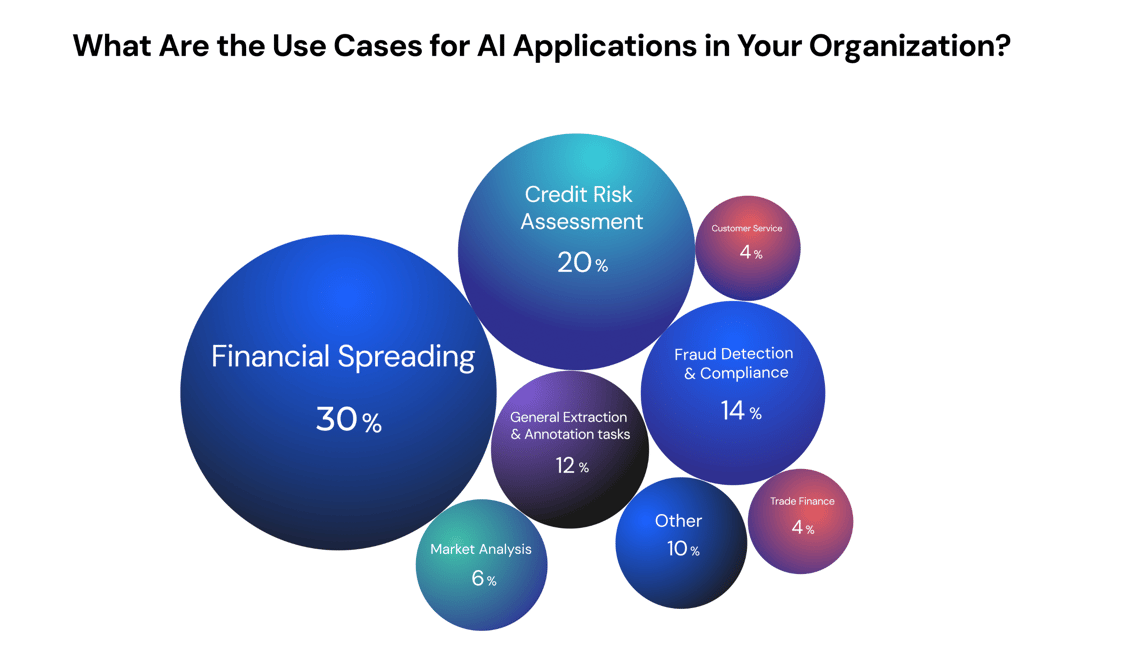
Financial spreading, or the automation of financial statement analysis, leads as a critical task, especially within corporate and institutional banking where time-consuming manual data processing has traditionally limited productivity.
The prioritization of fraud detection, a vital function in the financial services industry, further demonstrates the industry's focus on security and compliance. These applications show how AI-driven solutions are instrumental in automating workflows, reducing human error, and safeguarding against fraud in real time.
Key Challenges to AI Implementation
Despite the clear benefits AI can offer, the survey identified several hurdles that organizations face in its implementation. Participants frequently pointed out issues such as explainability of AI decisions, data privacy concerns, and challenges with integrating AI into existing systems. These are consistent with broader concerns across industries that deal with high-stakes decision-making.
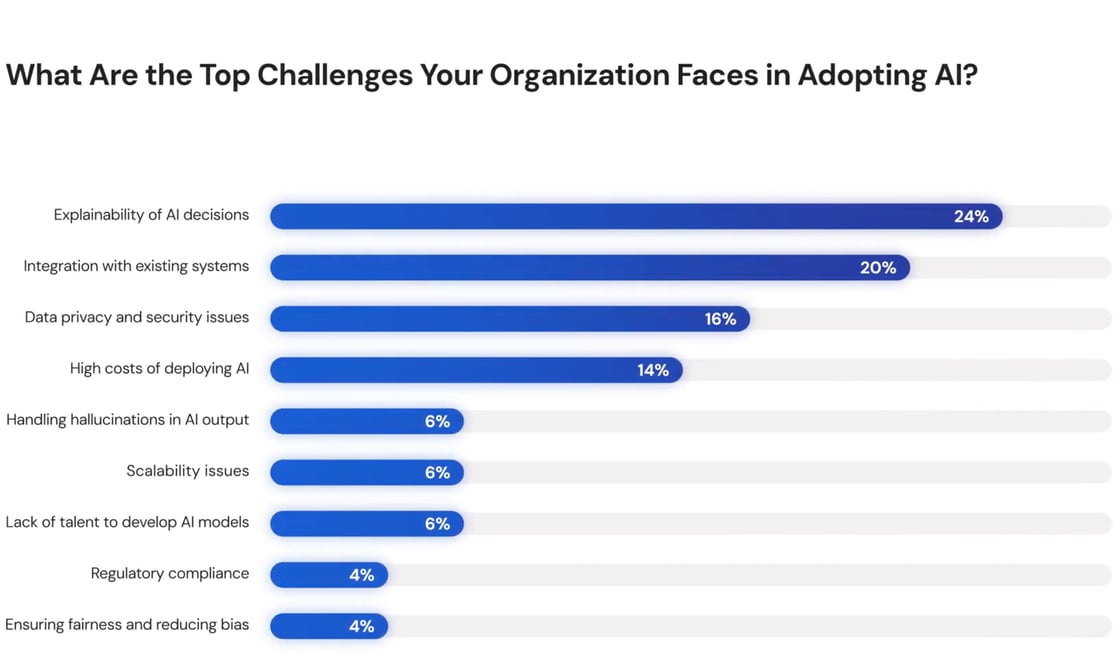
The challenge of explainability reflects the need for transparency in AI decision-making processes, particularly in sectors where human oversight and regulatory compliance are essential.
Furthermore, data privacy and security are primary concerns, especially in the financial sector, where safeguarding sensitive customer information is a top priority. Challenges in integration with legacy systems also underline the complexities many organizations encounter when adopting new technologies. These obstacles reveal that while AI is capable of advancing operational efficiencies, there is a need for robust and transparent AI frameworks that respect data privacy and integrate seamlessly with existing infrastructures. Only then can organizations achieve the full potential of AI.
Document Management: High Volume, High Accuracy Demands
When it comes to document-related tasks, respondents reported high-volume data extraction, classification, and validation as their primary needs. The frequent mention of such tasks highlights the need for advanced AI solutions that can handle vast amounts of information accurately and rapidly. Industries with stringent document management requirements, such as banking, insurance, and legal services, can particularly benefit from AI tools capable of
- extracting unstructured data with minimal error,
- classifying documents for easier retrieval,
- and validating data for downstream usability.
Document processing and management can become bottlenecks without effective AI tools that are designed to scale with organizational needs. The findings underscore the increasing demand for AI systems that can query and process large volumes of data simultaneously, enhancing not only speed and efficiency but also accuracy in data management tasks.
Investment in AI Expertise: A Collaborative Approach
The survey results indicate that many organizations are pursuing a collaborative approach to AI expertise, relying on internal AI teams as well as external partners, such as strategy consulting firms and big tech companies. This blend of internal and external resources reveals a pragmatic approach to building AI capabilities: organizations are looking to develop in-house expertise while simultaneously benefiting from the experience of established technology providers.
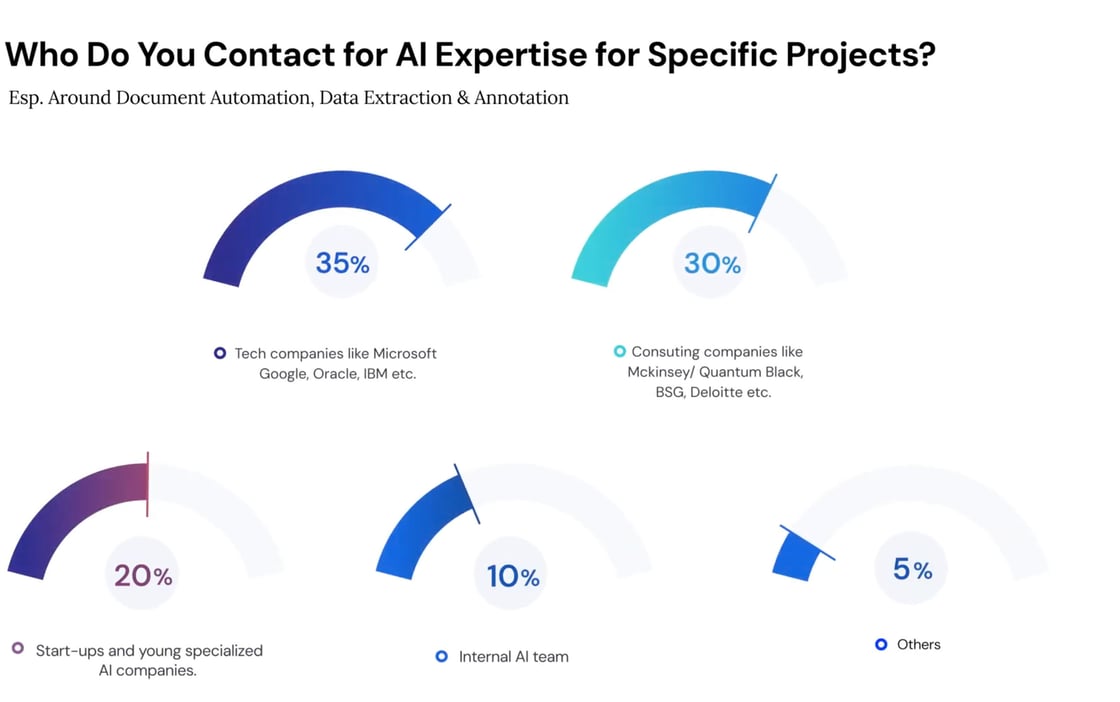
Especially, financial institutions looking to enhance use cases like financial spreading or credit risk assessment may find it beneficial to use external vertical AI expertise to handle industry-specific requirements effectively, more so since LLMs are playing a crucial role with their significant need for computational resources.
Addressing Explainability and Compliance Through AI Transparency
One of the most pressing challenges highlighted in the survey is the need for explainable AI. Explainability allows stakeholders to understand how AI systems make decisions, which is crucial in high-stakes environments like finance, where regulatory compliance and accountability are essential.
Incorporating mechanisms to trace lineage—understanding the source and transformation of data throughout its lifecycle—can help establish a clear audit trail and reinforce trust in AI-generated outputs. The ability to integrate domain-specific definitions and ontologies within AI models is another valuable practice for enhancing explainability, as it ensures that AI systems operate within the frameworks and expectations of the industry.
These survey insights emphasize that while explainable AI is an emerging field, it is quickly becoming non-negotiable. Organizations that prioritize explainability are more likely to see long-term success as they continue to deploy AI solutions at scale.
Conclusion: AI’s Role in the Future of Financial and Data-Intensive Sectors
The 2024 AI survey reveals a picture of high potential and clear challenges. Sectors like financial services, characterized by high volumes of unstructured, often layout-heavy data and rigorous compliance standards, are already benefiting from AI but face obstacles in implementation, including privacy concerns, explainability, and integration challenges. As organizations navigate these complexities, they require AI solutions capable of processing and querying substantial data volumes while ensuring data privacy and regulatory compliance.
For organizations focusing on AI-driven document automation with enhanced downstream usability, solutions that support real-time querying, high-volume data processing, and a privacy-by-design approach are becoming essential. Likewise, the demonstrated demand for financial spreading expertise shows the need for specialized AI solutions that can streamline financial reporting and analysis with accuracy and efficiency. In tackling explainability challenges, the ability to trace lineage and inject industry-specific definitions and ontologies can enhance model transparency and foster trust in AI-driven insights.
As these insights demonstrate, the future of AI in data-intensive sectors like financial services hinges on balancing operational efficiency with a transparent, responsible approach. With a deep focus on privacy, transparency, and financial expertise, companies dedicated to refining AI solutions for these specific needs are positioned to lead the next wave of AI-driven transformation.
- June 2025 (2)
- May 2025 (2)
- April 2025 (4)
- January 2025 (1)
- December 2024 (2)
- November 2024 (3)
- October 2024 (1)
- September 2024 (1)
- August 2024 (1)
- July 2024 (1)
- June 2024 (3)
- May 2024 (2)
- April 2024 (3)
- March 2024 (2)
- February 2024 (1)
- December 2023 (1)
- November 2023 (3)
- October 2023 (3)
- September 2023 (1)
- July 2023 (1)
- June 2023 (1)
- May 2023 (3)
- April 2023 (2)
- February 2023 (1)
- January 2023 (1)
- December 2022 (2)
- August 2022 (1)
- July 2022 (2)